Jan. 11, 2022
Machine learning tool increases accuracy of diagnosis in Parkinson’s disease

A new study is looking at a tool revolutionizing the collection of digital data. Local topological recurrence analysis (LoTRA) applies machine learning approaches to examine data from those individuals living with movement disorders, such as Parkinson’s disease.
The tool, designed by the Cumming School of Medicine Optogenetics Core Facility and CaPRI researchers (Calgary Parkinson Research Initiative) is a simple machine learning model capable of outperforming deep-learning models in detecting Parkinson’s disease from digitized handwriting samples. While deep-learning techniques have expanded the possibilities to facilitate the integration of decision-support systems into clinical medicine, they are associated with added computational complexity, the need for large datasets, and can have an astounding ecological effect in terms of carbon footprint.

Taylor Chomiak
Kelly Johnston
LoTRA significantly improves the computational efficiency of data analysis, decreasing the potential need for mass amounts of computing power to process data. LoTRA is based on the idea that data has “shape,” and that recurring patterns embedded in this shape can be identified. This new method of data analysis means that data could easily be analyzed by simple wearable/portable digital devices like a tablet, as more complex technology and infrastructure may not be needed for the same result.
"This represents an important step forward in making data analysis accessible,” says lead author Dr. Taylor Chomiak, PhD.
Especially for rural communities, the ability to process complex data efficiently where there may be limited infrastructure and internet connectivity, has the potential to be a game-changer for telehealth.
Through LoTRA, the CaPRI team can identify “digital biomarkers" — hidden patterns embedded in digital data, which, when identified, allow for more explainable AI-enabled diagnostic decisions, as well as the ability to non-invasively track the progress and effectiveness of interventions administered by clinicians. Digital biomarkers are also highly advantageous due to their ease of integration of data collection devices into daily life, their non-invasive highly quantitative data collection capabilities, and low cost.
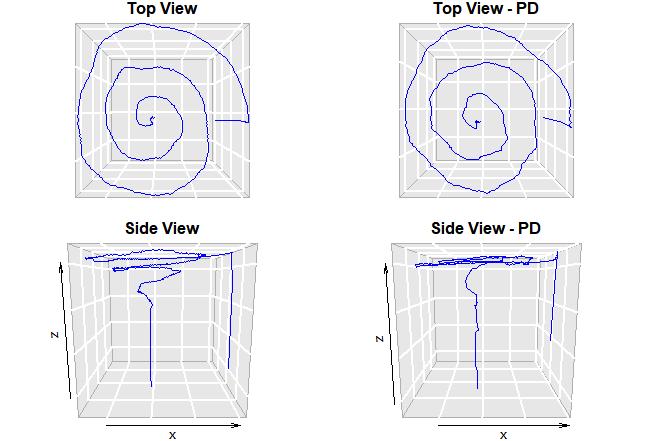
A sample of the digitized handwriting test, indicating both surface-level data as well as pressure (depth) data. Data is from the Parkinson Disease Spiral Drawings Using Digitized Graphics Tablet Data Set.
UC Irvine Machine Learning Repository
In the study, published in Nature’s Parkinson’s journal npj Parkinson’s Disease, the team used previously collected data of a simple handwriting test. Based on the digital biomarkers in the dataset, they were able to accurately predict the presence of Parkinson’s disease through computer-assisted analysis, all from an examination of a single digitized handwritten spiral drawing test. Unlike traditional paper and pencil writing tests, digital testing can allow for more information to be captured like writing pressure in addition to the x-y co-ordinates that can be used to help identify unique data shapes.
Deep-learning techniques generally require large amounts of data points to provide accurate results; thanks to the identification of digital biomarkers via LoTRA, we may now be able to draw the same conclusions with less data, in a shorter amount of time, at lower cost.
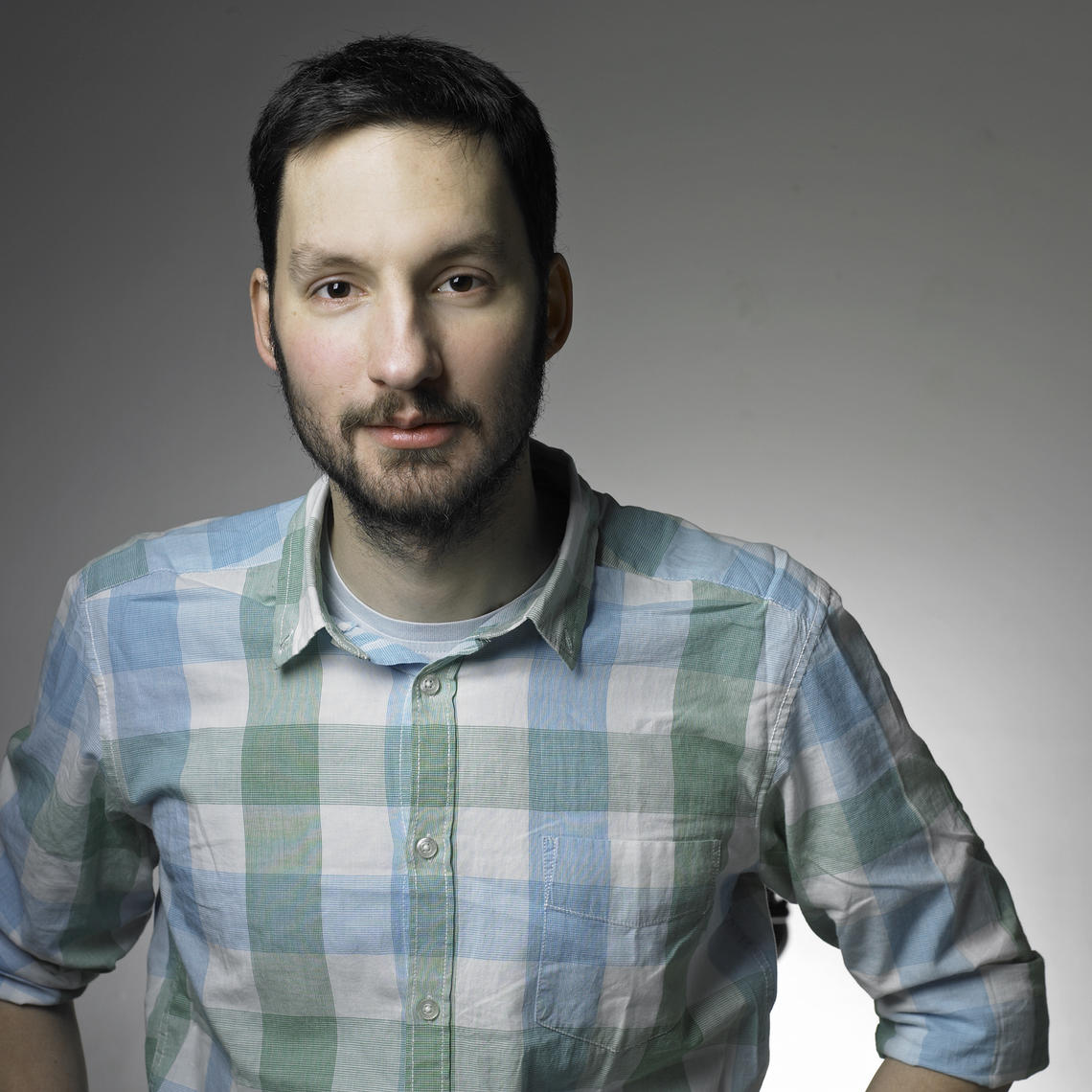
Tamás Füzesi
Courtesy Tamás Füzesi
“With a tool like ours, we receive not only enough data, but high-quality data, too,” says research scientist Dr. Tamás Füzesi, PhD. “The tool allows us to make improvements on data interpretation without needing to make improvements to the methods of data collection.”
The results from this study are laying the groundwork for a new generation of AI and machine learning assisted analysis, which will lead to fewer tests needed for the same or more accurate results. This will lead to more efficient diagnosis and more accurate treatment interventions for those individuals living with Parkinson’s disease. The tool also shows promise for use in analysis of other disorders and datasets as well.
Taylor Chomiak is a staff scientist at the Cumming School of Medicine Optogenetics Core Facility and adjunct assistant professor in the Department of Clinical Neurosciences at the Cumming School of Medicine at University of Calgary, and a member of CaPRI and the Alberta Children’s Hospital Research Institute.
Tamás Füzesi is a staff scientist at the CSM Optogenetics Core Facility, and a member of the Hotchkiss Brain Institute.
The Calgary Parkinson Research Initiative is based out of the Movement Disorders Clinic in Calgary and works closely with the Hotchkiss Brain Institute’s Movement Disorders Team at the University of Calgary. The Movement Disorders Clinic is hailed by the U.S. Parkinson’s Foundation as a Centre of Excellence, and the team runs clinical trials to deliver the best in available and centralized care to patients in Southern Alberta.